- Google researchers have developed a scent-describing artificial intelligence
- It predicts how a particular molecule will smell based on its chemical structure
- By plotting scent profiles on a map, it visualises how similar two molecules smell
- The team hope it can be used to identify new fragrances and insect repellents
Computer scientists at Google have developed an artificial intelligence (AI) tool that can describe what something will smell like by its chemical structure.
It uses an ‘odour map’ to visualise the indicative scents of a particular molecule, building on work from 2019 where the technology described scents using words.
Points that represent similar odours appear close together on the map, which can be used to predict what a substance will smell like before humans have a sniff.
‘The model is as reliable as a human in describing odor quality,’ the researchers from Cambridge, Massachusetts, USA wrote.
They hope that the AI model could be used to identify new scents for fragrances, or flavour profiles in food formulation.
It may even be able to suggest new, effective repellents for mosquitoes or other disease-carrying insects.
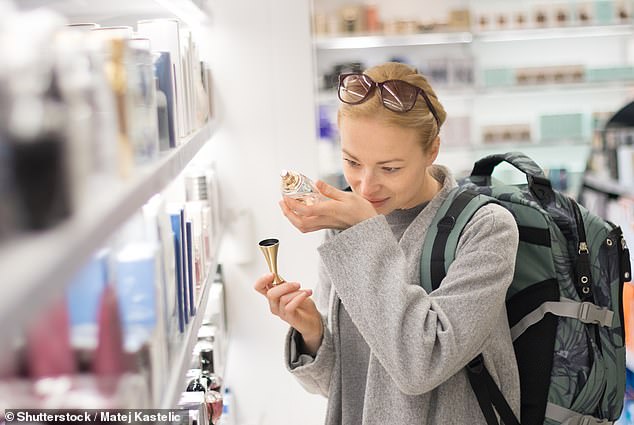
The AI uses an ‘odour map’ to visualise the indicative scent labels of a particular molecule. Points that represent similar odours appear close together on the map, and it can be used to predict what a substance will smell like before humans have a sniff
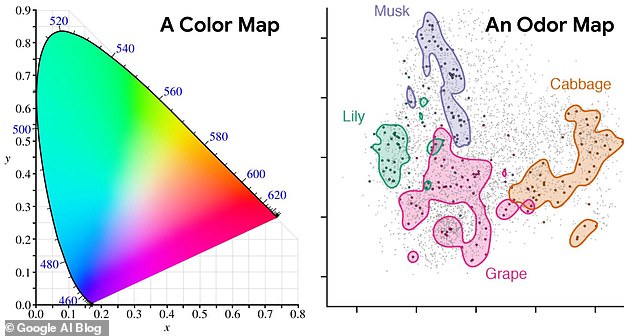
Left: An example of a colour map where locations give values for hue, saturation and wavelength of light. Similar colours lie near each other. Right: The Principal Odour Map. Individual molecules correspond to points (grey), and the locations of these points reflect predictions of their odour character
Mapping the range of scents perceptible to our noses is more difficult than, say, colours our eyes can pick up.
This is because the cone sensors in our eyes can only pick up three colours – red, blue and green – whereas we have more than 300 equivalent scent receptors.
This means that an individual can perceive a hugely diverse range of smells, and that there is an even larger range of smell capacities people could possess.
We also have subjective opinions on what things smell like, as there is a lack of any defining scents that are known to smell the same for all.
The Google team trained a neural network using flavour and fragrance datasets of over 5,000 different molecules, which resulted in the new ‘Principal Odour Map’ (POM).
In a paper published this month in bioRxiv, the researchers outlined three tests they performed with the Principal Odour Map to probe its scope.
To test the AI’s accuracy, they asked a panel of 15 people to describe the smell of 320 molecules that it had not been trained on.
As individuals perceived each odour slightly differently, the average of all the panellists was taken and compared to the AI’s results for these molecules.
‘We found that the predictions of the model were closer to the consensus than the average panelist was,’ the researchers wrote in a Google blog post.
‘In other words, the model demonstrated an exceptional ability to predict odor from a molecule’s structure.’
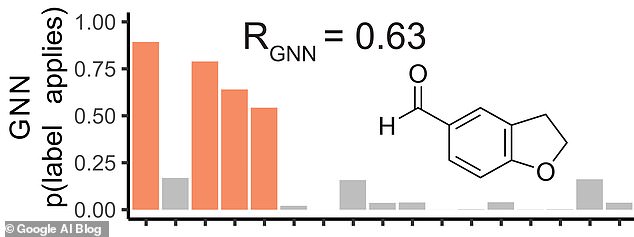
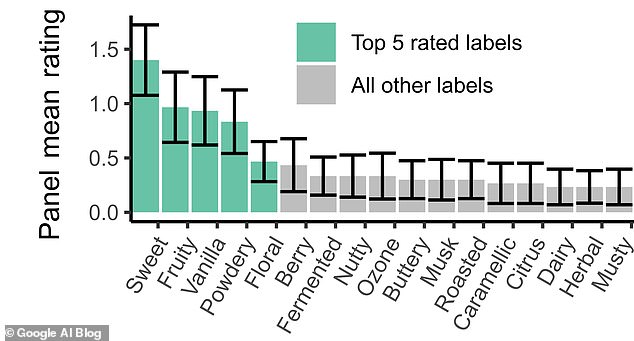
Top: Prediction of scent profiles by Google’s AI model for the molecule 2,3-dihydrobenzofuran-5-carboxaldehyde. Bottom: Average ratings given by trained panellists for the same molecule. Each bar corresponds to one odour character label. The model correctly identifies four of the top five, with high confidence. R = Prediction’s correlation to the full set of 55 labels
The AI was also able to accurately detect the smell strength and its similarity to other odours, as well as how it could be perceived by other animals.
The researchers said: ‘We found that the map could successfully predict the activity of sensory receptors, neurons, and behavior in most animals that olfactory neuroscientists have studied, including mice and insects.’
For the latter, they collected data on how different species perceive molecules that represent ‘metabolic states’ – or metabolites – like ripe or rotten, nutritious or inert and healthy or sick.
They found that, if a long series of metabolic reactions is required to turn one metabolite into another, then they would appear very far apart on the map.
In contrast, odorous metabolites that are very similar and appear close together only require a few metabolic reactions to be converted into one another.
This mirrors the evolutionary theory that animals’ ability to smell helps them clearly distinguish between these metabolic states.
‘The POM shows that olfaction is linked to our natural world through the structure of metabolism and, perhaps surprisingly, captures fundamental principles of biology,’ said the researchers.
It is hoped this information could enable the model to detect human and animal diseases.
In a third test, the team wanted to see if their AI could identify molecules that would work as mosquito repellents.
They retrained the neural network using two datasets that describe how well a given molecule can keep mosquitoes away.
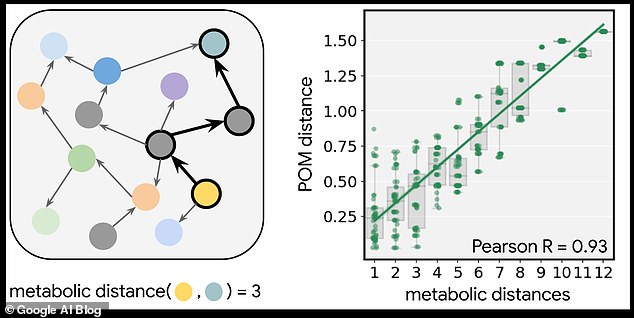
Left: Illustration visualising metabolic reactions found in 17 species across 4 kingdoms. Each circle is a distinct metabolite molecule and an arrow indicates that there is a metabolic reaction that converts one molecule to another. Metabolites without an odour are shown in grey. The metabolic distance between two odorous metabolites is the minimum number of reactions necessary to convert one into the other. In the path shown in bold, the distance is 3. Right: Metabolic distance was highly correlated with distance in the POM
It was found to be able to predict the mosquito repellency of nearly any molecule, including ones not from the datasets that were validated experimentally.
The researchers wrote: ‘We…found over a dozen of them with repellency at least as high as DEET, the active ingredient in most insect repellents.
‘Less expensive, longer lasting, and safer repellents can reduce the worldwide incidence of diseases like malaria, potentially saving countless lives.’
The same method could be applied to find molecules that repel other disease-carrying organisms in future.
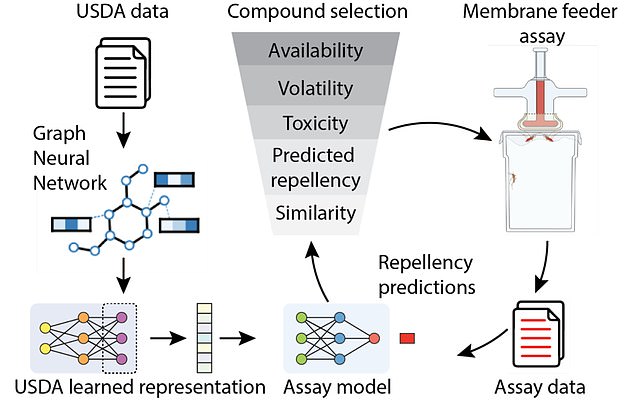
The researchers retrained the neural network using two datasets that describe how well a given molecule can keep mosquitoes away. One was from the USDA, and the other used laboratory mosquito feeder assay data, which was used iteratively to improve predictions.
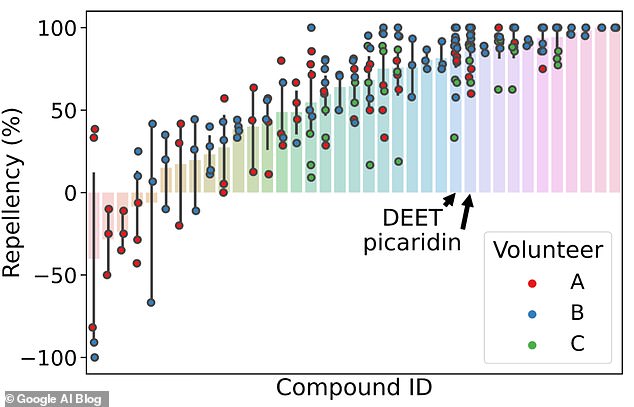
The model identified many molecules that showed repellency greater than the most common repellents used today (DEET and picaridin)
Some scientists, however, are sceptical about the efficacy of the AI, claiming it does not look at how the human brain receives and translates scent information.
The work also does not account for smells that are the result of a complex combinations of scent molecules.
Barry Smith, from the School of Advanced Stud at the University of London, told New Scientist: ‘Nearly all of the smells we are aware of – wine, coffee, soap, other people, the sea – are due to a mixture of several hundred volatile molecules.
‘Eating food, there’s the saliva in our mouths, there’s the taste receptors contributing, the texture of the food.
‘Many things are interacting to give you a multi-sensory experience. So I think we are still far away from simply predicting flavour from food molecules.
‘We will still have to fill in the biology eventually if we want to understand how humans perceive odours.’
Source: Google AI is as reliable as a human at describing what things smell like | Daily Mail Online